How is bias built into algorithms? Garbage in, garbage out.
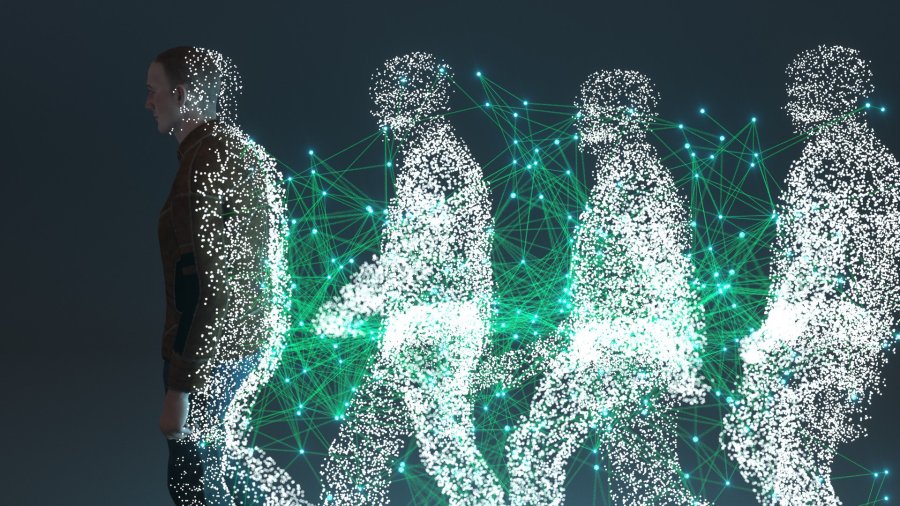
In facial recognition and AI development, computers are trained on massive sets of data, millions of pictures gathered from all over the web. There are only a few publicly available datasets, and a lot of organizations use them. And they are problematic.
I spoke with Vinay Prabhu, chief scientist at UnifyID. He and Abeba Birhane, at University College Dublin, published a paper recently examining these academic datasets. Most of the pictures are gathered without consent, people can be identified in them and there are racist and pornographic images and text. And even the idea of labeling someone a lawyer or a woman or a criminal based on appearance? Well. Ultimately, the researchers said maybe it’s not the data that’s the problem. Maybe it’s the whole field. The following is an edited transcript of our conversation.

Vinay Prabhu: The community has a historical prior of basically pursuing problems which are ethically dubious. A huge number of papers are published on ethnicity classification and generating human faces and basically ranking people’s faces as to how attractive it is. I mean, is there really a need to be solving these problems in the first place, what exactly it is that you’re trying to automate? Ask yourself, what is your technology eventually going to result in? How is it going to result in terms of shifting of the power in the society? The computer-vision community has a deeply entrenched historical track record of basically increasing the wrath of power on the minority groups. If you’re looking at the flagship applications, there are very few things that have ushered in a paradigm shift in the way that disenfranchised people have now felt enfranchised.
Molly Wood: It sounds to me what you’re saying is, don’t just design a better image-based dataset. The idea that you need an image-based dataset, and that technology should be built on top of that data, is itself flawed and will always be flawed?
Prabhu: You hit the nail on the head. Women of color have done tremendous work, but then every time they try to do something good, the tech boys or the tech bros, will invariably attack them as social justice warriors who are bringing their cancel culture into academia. Whereas, we need to be more pragmatic. We need to be more science-oriented. We need to be oblivious to all of these politics, is what their excuse is.
Wood: There are conversations about banning facial recognition technology that’s being developed in these ways. Is this a problem for regulation to solve?
Prabhu: Regulation is certainly required, but if you pass a legislation, it’s pretty easy to discover a loophole. I think one of the Silicon Valley cliches is, if you don’t allow us to loot the data from the public, China is doing the same thing. Russia is doing the same thing. Their AI will basically be superior to ours. Legislation, I think, will for the most part put a small roadblock. But I am very confident of the ability of the powers that be to find loopholes and to harness solutions that will allow them to still stay within the legal realm.
Related links: More insight from Molly Wood
Birhane and Prabhu’s research paper about computer vision, datasets and algorithmic development reads surprisingly like philosophy. The paper notes that the researchers only examined publicly available and well-known datasets where the source of most of the images is relatively known, like Flickr or Google image search. But, say the writers, “current work that has sprung out of secretive datasets, such as Clearview AI, points to a deeply worrying and insidious threat not only to vulnerable groups, but also to the very meaning of privacy as we know it.”
MIT operates one of the public datasets that Birhane and Prabhu examined. In response to their research, MIT took it offline for good. That dataset had about 80 million images. Google trains machine learning algorithms on a fairly secretive dataset called JFT-300M, which has a reported 375 million images that came from somewhere. By comparison, Clearview AI has scraped more than 3 billion images off the internet and is using them to train facial recognition algorithm technologies to sell to law enforcement. And we have no idea what’s in there. There’s a piece in the Atlantic from July 5 called “Defund facial recognition.”
Also watching:
The results of an independent audit of Facebook by civil rights researchers found that the platform and the policies of the company are not good for civil rights. The auditors found that the speech issues on Facebook and its other properties are significant, and its refusal to police political speech and misinformation has serious real-world impact and could end up affecting the November election. Facebook says that its oversight board, which was proposed in 2018 to supposedly provide some oversight over content decisions made inside the company, not only won’t have anything to do with misinformation that could affect the election, it probably won’t actually be formed until after the election.
The future of this podcast starts with you.
Every day, the “Marketplace Tech” team demystifies the digital economy with stories that explore more than just Big Tech. We’re committed to covering topics that matter to you and the world around us, diving deep into how technology intersects with climate change, inequity, and disinformation.
As part of a nonprofit newsroom, we’re counting on listeners like you to keep this public service paywall-free and available to all.
Support “Marketplace Tech” in any amount today and become a partner in our mission.