The hidden meanings of the AI industry’s favorite words
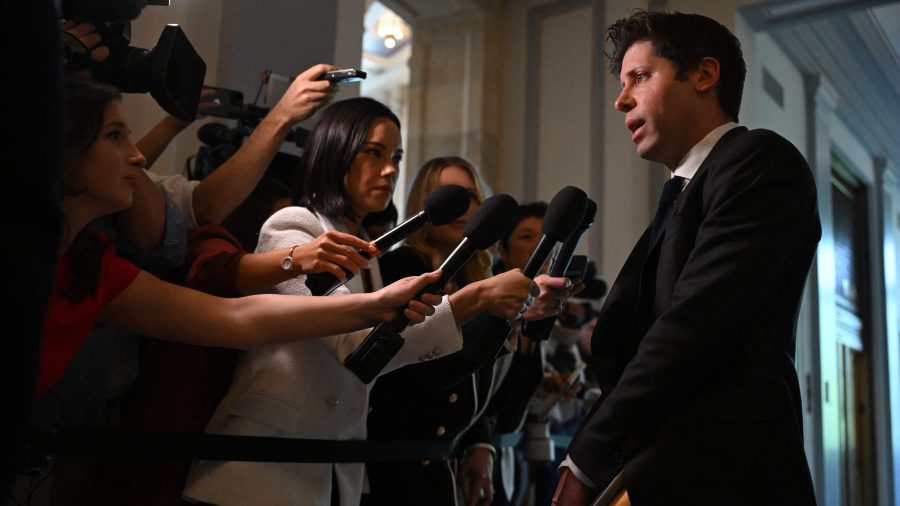
We hear words like “safety” and “transparency” thrown around a lot in the AI industry, but they don’t always mean the same things to tech insiders that they do to the rest of us.
Take, for instance, a 2016 paper titled “Concrete Problems in AI Safety,” written by a roster of prominent artificial intelligence researchers who have OpenAI, Google and Anthropic on their resumes. As tech journalist Karen Hao, a contributing writer to The Atlantic, points out, the “safety problems” described in that paper eight years ago may not be the same safety problems that consumers or policymakers are most worried about.
Tech leaders are concerned about the risk that machine learning systems could behave in unintended ways. But when technology users and policymakers talk about safety, they’re often referring to violations of privacy, bias, disinformation, risks to children online and other actual harms to humans.
Hao wrote a glossary of terms like this a few years ago for MIT Technology Review called “Big Tech’s guide to talking about AI ethics.” Marketplace’s Meghan McCarty Carino spoke with her about some of the biggest double meanings on her list.
The following is an edited transcript of their conversation.
Karen Hao: When we talk about safety in the public domain, you automatically think, oh this system isn’t going to harm me. It’s not going to violate my privacy. It’s not going to make judgments on me that could greatly negatively impact my life. But in the “Concrete Problems in AI Safety” paper, it explicitly says that none of these things are what they mean by “safety.” For them, it just refers to essentially preventing AI from becoming rogue and misaligned. Of course, because of this confusion, companies can say we are investing a lot in AI safety, and we care a lot about keeping AI safe. And that does a lot of work for them because the public is assuming that all of these things are taken care of.
Meghan McCarty Carino: What are some of the AI harms that the public might be thinking of and that they would like to be protected from?
Hao: One of the things that we’ve seen a lot of research done recently, both in, in academia and in journalism, is how image generators can create really racist, stereotypical images. These kinds of embedded biases have a really big impact on the content that we’re going to continue seeing on the internet because these systems are being used to generate enormous volumes of images and text. And that content goes back into the internet and into downstream applications that are being built on top of these technologies. That is one of the very prevalent, glaring harms that we see now and that companies are not really talking about and not investing a lot into changing.
McCarty Carino: What’s really at stake here? Why is it important that the industry is using language in a way that might be at odds with how the public or policymakers interpret it?
Hao: I think what’s at stake is just that we won’t realize what the actual harms are. If companies can use terms to shift our focus to a different set, to look somewhere else, they get to continue doing what they’re doing elsewhere. And so, what’s at stake is that we are going to end up with these terminologies and these definitions codified into the regulatory frameworks that are meant to hold these companies accountable. And in fact, it ends up just paving the way for them to continue doing what they’ve always done, which could also involve harming the public.
McCarty Carino: Back in 2021, you put together this glossary of some of the common terms that come up a lot in AI ethics. “Safety” is one of them, which we just talked about, but we wanted to dive into some others. Let’s start with the word “transparency.” This sounds like a good thing. Do you want to read the definition of “transparency” that you wrote?
Hao: Yeah, this was one of my favorites at the time. “Transparency, a noun. Revealing your data and code. Bad for proprietary and sensitive information. Thus, really hard; quite frankly, even impossible. Not to be confused with clear communication about how your system actually works.”
The thinking behind this one was, there were so many arguments that I was hearing at the time from these companies saying, we can’t actually do what you’re asking us to do, and we can’t just publish our code on the internet. An even subtler one was when Elon Musk was buying Twitter and he said we will publish the code, which is also very interesting. It’s like two sides of the same coin, where automatically in both instances, there is the equating of transparency with the literal publication of code, which, if you think about it, the average person in the public doesn’t actually know how to read code. So that doesn’t actually increase transparency at all. The way that AI systems work is not present necessarily in the code. The code just says we’ve digested this data. And that’s all it says. It doesn’t say what the machine has learned. It doesn’t say how it’s making decisions. That actually all has to be audited through other means other than just reviewing code. And so, it’s one of those examples where the tech industry can easily turn the definition of one word that means something totally different in the public domain into something else that then becomes intractable.
McCarty Carino: We also have to talk about the word “regulation.” This is something that comes up a lot in this context. What do industry leaders mean when they say “regulation”?
Hao: The thing that companies always do is they call for regulation as a way to kind of shift responsibility of mitigating the harmful impacts of their technologies to policymakers. And it’s sort of a common saying within Silicon Valley that government and policymakers are incompetent. And they believe that the government will actually ultimately be ineffective and not be able to implement this regulation. But if you, if you say, oh, yes, we do want to work with you to develop this regulation, then you seem like the nice guy that is trying to cooperate. And this is exactly the way that OpenAI does it. Sam Altman said in his testimony to Congress that we believe regulating AI is essential, we’re eager to help you, we’re going to work with you to balance the harms and benefits of this technology. But then, as you can see in just the past few months, there has been significantly more pressure from different groups like artists associations or from authors and journalism organizations demanding some kind of regulation around the use of copyrighted material in training AI systems. And now, OpenAI sort of changes their tune. They say, because copyright today covers virtually every sort of human expression, including blog posts, photographs, forum posts, scraps of software, code and government documents, it would be impossible to train today’s leading AI models without using copyrighted materials. So, what that suggests is, before, when OpenAI was talking a lot about how they would love regulation, it means they want to shape regulation that will entrench their dominance rather than check it. And then when you, when push comes to shove, you realize their real intent, or, like, the real meaning behind the way that they use this term is just as a shield, rather than to truly collaborate with government.
McCarty Carino: You wrote your AI glossary back in 2021, at the dawn of the AI boom and before ChatGPT really exploded in the public eye. Are there any terms that you would add to that glossary now, almost three years later?
Hao: Yeah. So, one thing that’s happened is the anthropomorphizing of AI systems has gone through the roof. One specific term that falls in this category is “hallucinations.” “Hallucinations” for the AI industry refers to when a generative AI system produces some kind of information that is incorrect or undesirable. The thing that I find problematic about this term is that it makes it sound like it is just an accident. When humans hallucinate, it’s like a weird aberration in our behavior. It is a weird anomaly. But in an AI system, it is literally designed to do that. And so, there’s this kind of subtle distortion through the term “hallucination” that suggests that somehow this is abnormal and it will be fixed at some point, and then the chatbots will start to behave normally, in a way that is expected. The underlying technology is designed to hallucinate, so we need to find sort of a better term for this.
There are also all these arguments that with the copyrighted data lawsuits, for example, that AI systems are “inspired” just like human artists, or they “read” just like humans read. And that is just wholly not how these systems work. They’re not reading like humans or being inspired like humans. But these are very, very clever ways of taking the legal liability off of these organizations that are just wholesale hoovering up data that they are legally not allowed to use. And so, I think there’s a whole category of those types of terms now that are used to continue to confuse what’s actually happening underneath the surface with the development of these technologies. They’ve become increasingly effective because so many people interact with ChatGPT and do feel like they are talking to some kind of human on the other side. And that’s why those terms are so persuasive.
Though she wrote her AI ethics glossary for the MIT Technology Review, Karen Hao is now a contributing writer at The Atlantic. She most recently wrote about the environmental impacts of the AI boom, which is fueling the growth of power- and water-hungry data centers in regions that are warming and struggling with drought due to climate change.
She’s also working on a book about the AI company OpenAI, which is due out next year.
The future of this podcast starts with you.
Every day, the “Marketplace Tech” team demystifies the digital economy with stories that explore more than just Big Tech. We’re committed to covering topics that matter to you and the world around us, diving deep into how technology intersects with climate change, inequity, and disinformation.
As part of a nonprofit newsroom, we’re counting on listeners like you to keep this public service paywall-free and available to all.
Support “Marketplace Tech” in any amount today and become a partner in our mission.