What happens when AI is entrusted with medical decisions?
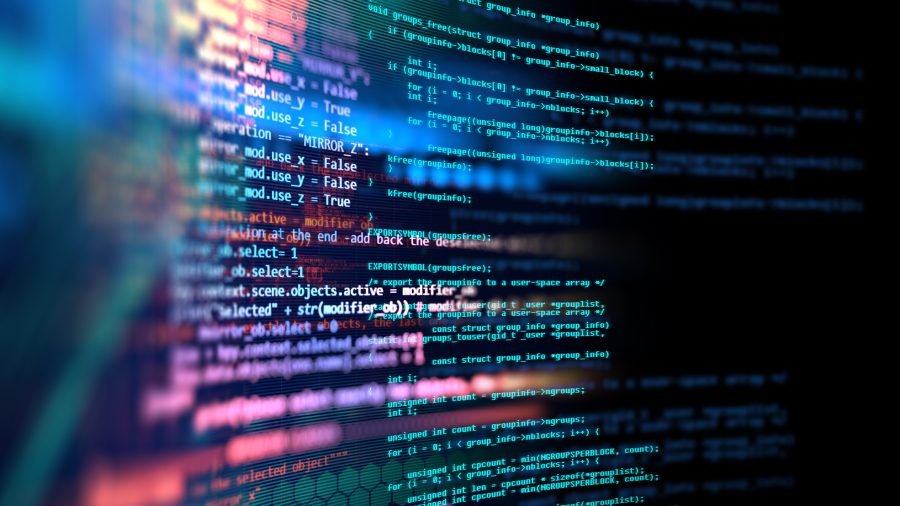
There’s a lot of excitement about how artificial intelligence is transforming health care, from diagnosing diseases to creating personalized treatment plans.
But just because AI can do something, doesn’t always mean it can do it better than a human, according to Meredith Broussard, a journalism professor at New York University and author of the book “More Than a Glitch: Confronting Race, Gender, and Ability Bias in Tech,” released last month.
Yesterday we featured part one of our discussion with Broussard, about how AI can magnify social harms. Today we continue that conversation, this time discussing what it means to entrust machines with our health.
Marketplace’s Meghan McCarty Carino spoke with Broussard about how that trust in machines is part of a broader tendency she calls technochauvinism.
The following is an edited transcript of their conversation.
Meredith Broussard: Technochauvinism is a kind of bias that says that technology or technological solutions are superior to others. People will say things like, “We should use an algorithm to decide who gets a kidney transplant because the algorithm will make an objective evaluation of who is most worthy of a donor organ.” Well, for many years, that algorithm was biased against Black people because of a racist assumption about Black bodies. That racist assumption got embedded in the math that was then used in every medical lab.
Meghan McCarty Carino: What would it look like to create an AI system without bias? Is it possible?

Broussard: I think it brings us back to this idea of the quality of the data that we’re using to feed an AI system in the first place. People often will look at something like a mortgage-approval algorithm that is biased and say, “Well, let’s just put in better data.” For example, there was an investigation in The Markup recently where they looked at mortgage-approval algorithms and found that these automated systems were 40% to 80% more likely to turn down a borrower of color than their white counterparts. Why is this? It’s because the mortgage-approval algorithm was fed with data about who had gotten mortgages in the past. Well, in the U.S., there’s a long history of financial discrimination. There’s a long history of redlining, of residential segregation. So, it’s unsurprising that the mortgage-approval algorithms are biased against people of color. People say, “Well, we can just make the mortgage-approval algorithm better by putting in better data.” Mathematically speaking, that is absolutely true, but there is no such thing as better data because we don’t live in a perfect world. We need to improve the world in order to improve the algorithm, and we need to not pretend that the algorithm is going to be better than what we have now.
McCarty Carino: In your book, you write about some of the many ways that artificial intelligence and algorithms have become embedded into policing, criminal justice and education. It almost seems like the more complex the problem, the more likely we are to turn to technology to try to solve it. What’s problematic about that approach?
Broussard: One of the ways I like to think about it is to divide these scenarios into easy problems and hard problems. We’re at this really interesting point right now where all of the problems that are easy to solve with technology have actually been solved, and so we’re only left with hard problems. Social problems have been going on for a really long time, so the idea that there would be an easy technological fix for them is a technochauvinist notion. You can’t take something like allocating public benefits and expect that you’re going to just write some code and fix the problem. In fact, code isn’t actually the solution in a lot of cases. For example, the problem of people being unhoused, we don’t need more code for that, we need houses for people.
McCarty Carino: You write a lot about the use of AI in medicine, and it’s something that you have some personal experience with.
Broussard: I had breast cancer during the pandemic, and one day I was poking around in my online medical chart, and I noticed a note that said, “an AI has read your scans.” I thought that was really weird. Why did an AI read the scans? What did it find? And after I recovered, I was still really curious, so I went on this journey of discovery where I took my own mammograms, and I ran them through an open-source AI in order to find out if that AI would detect my cancer, but also to write about the state of the art in AI-based cancer detection.
McCarty Carino: This provides an interesting illustration of the differences in how human brains work and how AI works. How are these processes different? And what do those differences tell us?
Broussard: The state of the art in AI-based cancer detection is not nearly as advanced as you might expect from what we see in media coverage and in Hollywood. I should say that the AI that I used did detect my cancer, but it did not do it in exactly the way that I expected. It’s not going in and saying, “Oh, I think you have this or that kind of cancer,” it’s just drawing a red box. That red box is an area that it has identified that the human doctor should follow up on. So, I think in general, we are pretty far from autonomous cancer diagnostic systems. It’s really still a human-in-the-loop process. I’m optimistic about the possibilities for AI assisting doctors in the future, but I am not optimistic about some shiny future where AI replaces doctors. I don’t think that’s realistic.
Related links: More insight from Meghan McCarty Carino
Last month, Wired published an excerpt of Broussard’s book from the chapter about her odyssey to understand her own breast cancer diagnosis and the role that AI played.
In another chapter of her book, Broussard writes about the research conducted by one of the most prominent voices speaking out about biased algorithms: Joy Buolamwini. She’s the founder of the Algorithmic Justice League and the subject of a documentary on Netflix called “Coded Bias.”
Buolamwini spoke to “Marketplace Tech” a few years ago about the research that put her in the spotlight, when she uncovered large racial and gender biases in facial recognition technology. Her study found the tech misidentified dark-skinned women more than a third of the time while with light-skinned men, its error rate was less than 1%.
The future of this podcast starts with you.
Every day, the “Marketplace Tech” team demystifies the digital economy with stories that explore more than just Big Tech. We’re committed to covering topics that matter to you and the world around us, diving deep into how technology intersects with climate change, inequity, and disinformation.
As part of a nonprofit newsroom, we’re counting on listeners like you to keep this public service paywall-free and available to all.
Support “Marketplace Tech” in any amount today and become a partner in our mission.